The journey of digital twin technology begins with the development of computer-aided design (CAD) software in the mid-20th century. The genesis of 3D CAD/CAM is often credited to French engineer Pierre Bézier, associated with Arts et Métiers ParisTech and Renault, following his groundbreaking mathematical research on surfaces. CAD revolutionized product design by enabling engineers and designers to produce accurate 2D and 3D representations of components and systems. Initially developed for the aerospace and automotive industries, CAD quickly expanded to encompass a wide range of applications, including architecture, electronics, and consumer products. CAD streamlines the design process, allowing for rapid prototyping, visualization of concepts, and precise documentation. Its widespread adoption has led to increased efficiency, reduced costs, and greater innovation across various industries.
As computing power advanced, so did the capabilities of simulation software. Finite element analysis (FEA) and computational fluid dynamics (CFD) emerged as powerful tools for simulating the behavior of complex systems, from structural components to fluid flow dynamics.
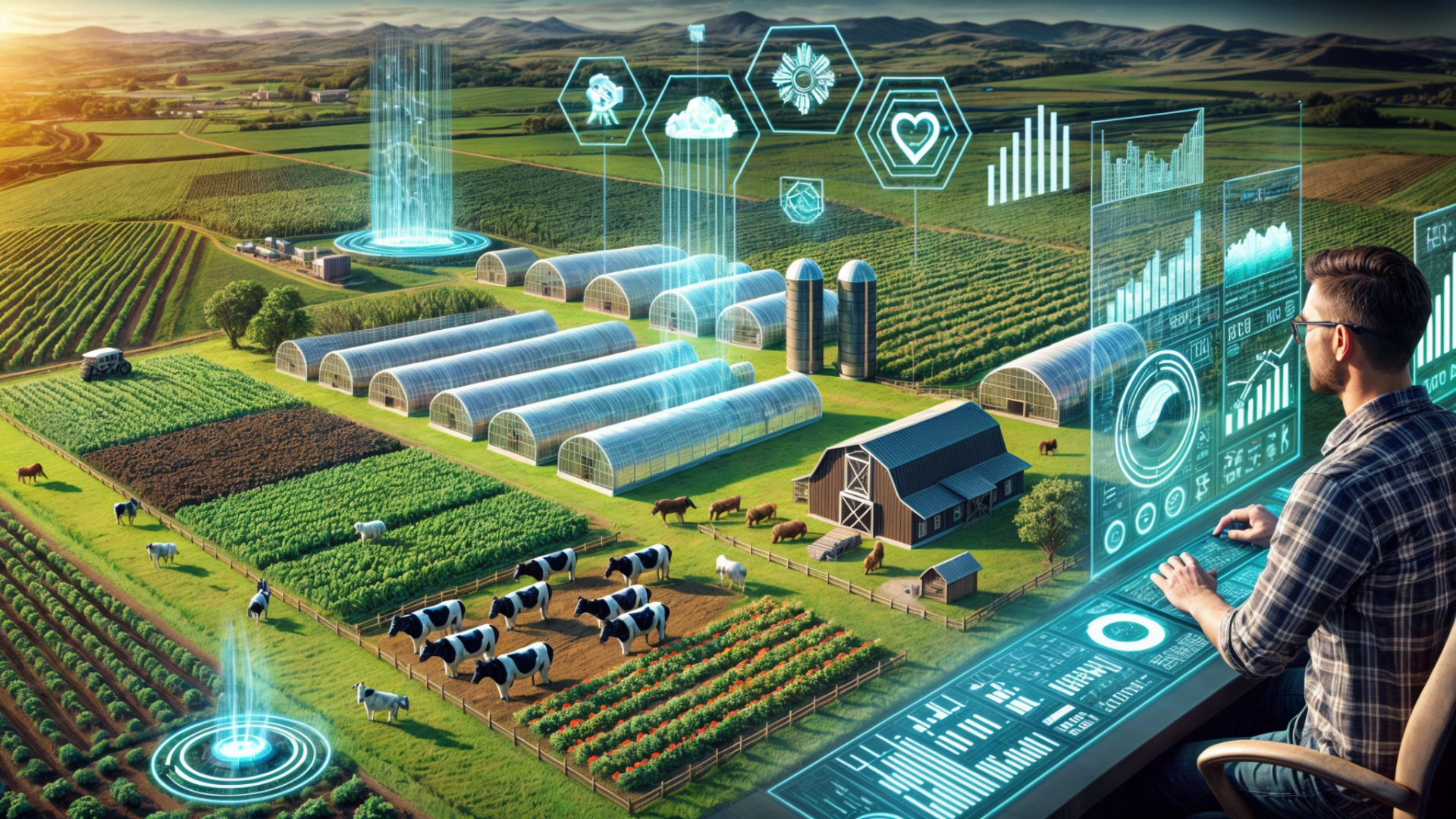
The concept of the digital twin truly began to take shape with the advent of cyber-physical systems (CPS) and the IoT (Internet of Things) in the late 20th and early 21st centuries. These interconnected networks of sensors, actuators, and computing devices paved the way for real-time data collection and analysis, laying the foundation for the creation of virtual replicas of physical assets. However, one of the earliest applications of digital twin technology can be traced back to NASA’s Apollo missions in the 1960s. This early use case demonstrated the potential of digital twins to improve safety, reliability, and efficiency in complex systems. This concept was a dynamic simulation tool for the Apollo missions. Following the oxygen tank explosion on Apollo 13 and the consequential damage to the main engine, NASA responded by deploying multiple simulators to assess the failure. These simulations evolved to incorporate digital components, creating the pioneering “digital twin.” This innovation allowed for the continuous integration of data to model the events leading up to the accident, enabling forensic analysis and informing future actions. From then NASA and the aerospace community continue to refine and utilize high-fidelity digital models to simulate physical systems and their operating environments.
In the decades that followed, digital twin technology found applications across a diverse range of industries. In manufacturing, digital twins are used to optimize production processes, predict equipment failures, and reduce downtime. In healthcare, digital twins of individual patients enable personalized treatment plans and predictive analytics for disease management. For example, digital twins can predict the electric activity in the heart of heart-rhythm disorders. The digital twin encompasses the structural composition of the heart, including any remodeling due to diseases such as damage, fibrosis, and inflammation, which are identified through techniques like MRI or PET scans.
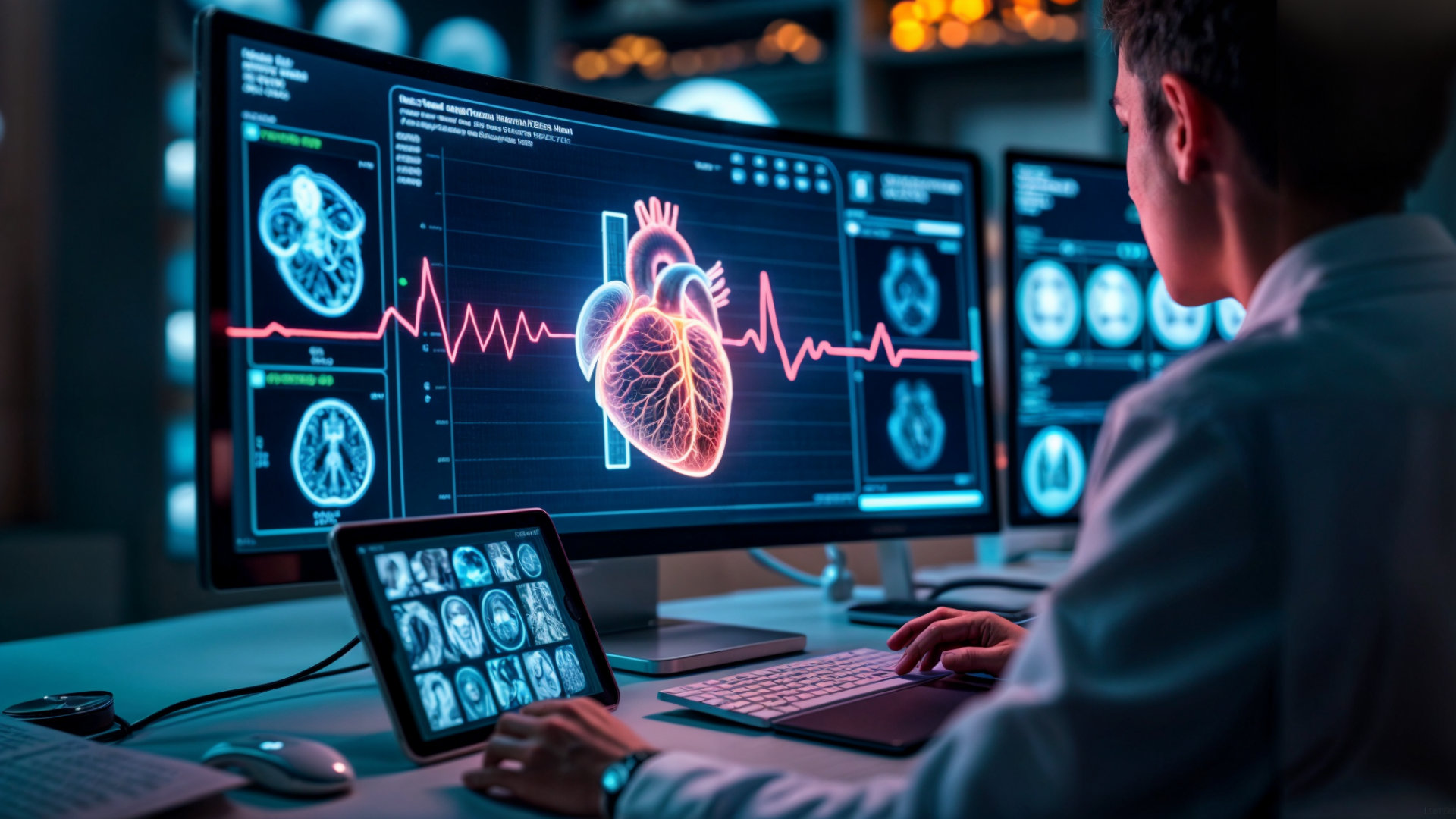
During normal function, an electrical wave triggers a contraction in the heart. However, if there’s scarring or damage present, the wave may get caught in that area. Instead of propagating through the heart, it recirculates, leading to arrhythmias. To effectively treat these arrhythmias, the digital twin must faithfully replicate both the structural damage and the electrical behavior of each heart cell. Using the digital twin, the doctors can send a signal and watch how the electrical wave propagates through the model. If anything is abnormal, it will likely present as an arrhythmia in the digital twin. This way, the arrhythmia can be predictable.
The potential applications of digital twin technology are virtually limitless. In urban planning, digital twins of cities can simulate traffic patterns, energy consumption, and environmental impacts to inform policy decisions and infrastructure development. In agriculture, digital twins of crops and livestock can optimize resource allocation, improve yield predictions, and enhance sustainability practices.
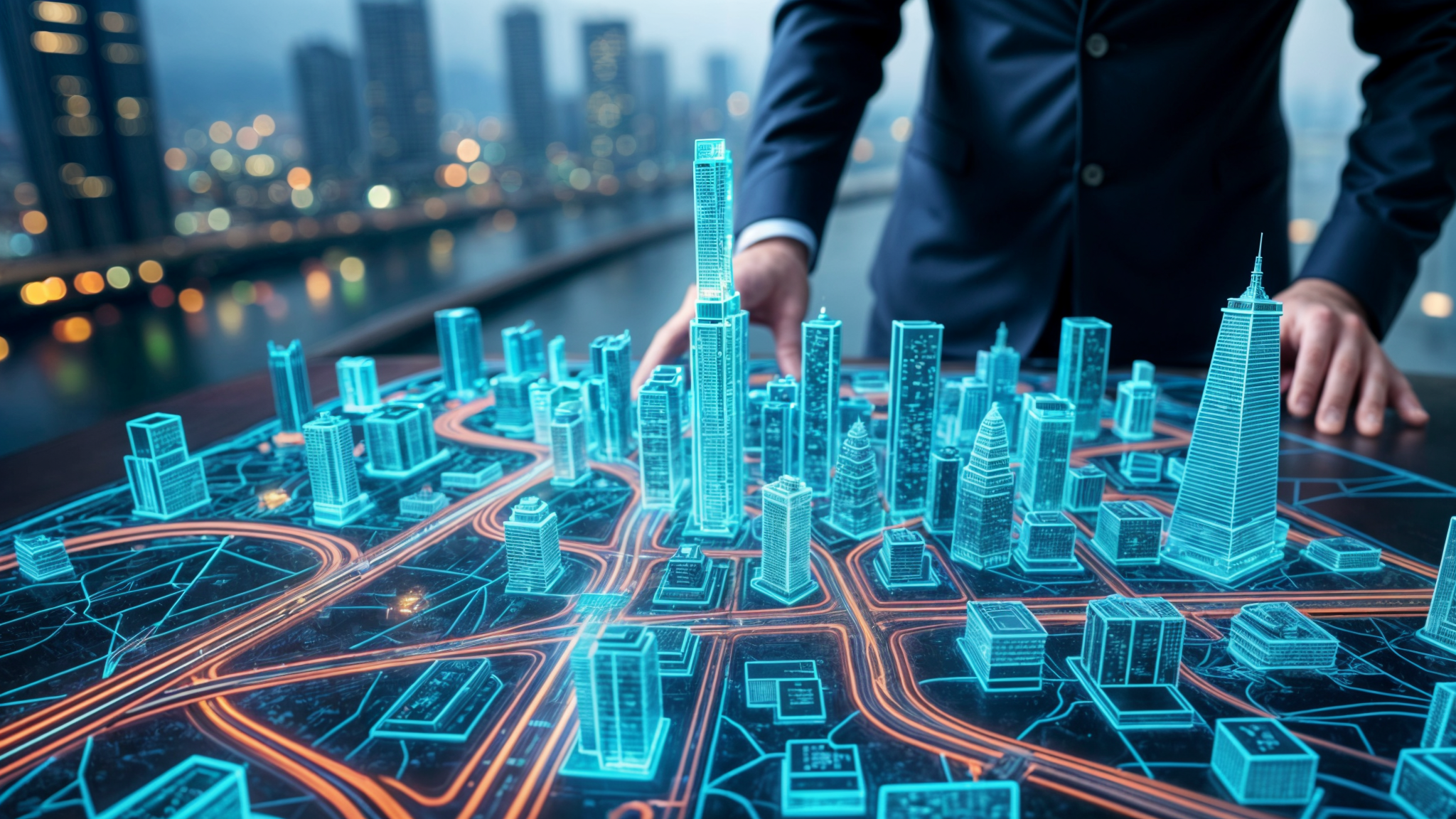
In the agri-food sector, these digital models can revolutionize production lines, making operations more efficient and cost-effective, while also enabling predictive analytics. Our podcast explores how Monitwin, AGRI-FOOD.AI’s digital twin model, can optimize the taste in breweries without the need for actual brewing. Additionally, vegetable-freezing facilities can utilize digital twin models to precisely define pre-cooking parameters before freezing, ensuring optimal product quality and efficiency.
Looking to the future, the development of advanced artificial intelligence (AI) and machine learning algorithms promises to further enhance the capabilities of digital twin technology. AI-driven digital twins will be able to autonomously analyze vast amounts of data, identify patterns, and make predictive insights, revolutionizing decision-making processes across industries.
Do you want to know more? Discover the 3rd episode of AGRI-FOOD.AI podcast – and get the picture!